Share this Post
The modern business is becoming increasingly data driven. “Data is the new gold!” leaders cry — and it’s true. By harvesting customer and business data, and then by cleaning it, processing it and analysing it, an organisation can gain hugely valuable insights into customer trends and business operations, and thereby make better decisions that drive efficiency and profitability.
And this is precisely why a powerful enterprise resource planning (ERP) system such as metasfresh is such a valuable tool — a sophisticated software solution that integrates an organisation’s financials, supply chain, operations, reporting, HR, product development and planning under a single information system. With metasfresh, businesses can effectively open up their own goldmine of data that can be leveraged for significant competitive advantage.
However, like gold, data isn’t valuable in its raw state. In the same way that gold first needs to be processed — minted into coins or fashioned into jewellery — in order to extract its value, so too does data. Indeed, it is in the business knowledge and insights that processed data reveals — rather than in the raw data itself — where the real value lies, and why more and more organisations are turning to data science to make sense of the information they have and use it to drive profitable actions.
The Great Transition — From Business Intelligence to Data Science
Traditionally, the goal of analysing business data has been to gain business intelligence (BI) — that is, to understand the business’s historical performance. In other words, BI is about looking to the past. Today, however, with the growth of analytics over recent years, organisations are now looking towards data science to peer into the future.
In essence, while BI asks, “What happened, and what do we need to change?”, data science takes things further, asking, “Why did this happen, and what may happen in the future?”
Rather than simply reporting on past performance, data science aims to predict future trends — and even offer prescriptions for success. While of course BI (i.e. a review of current facts) is indeed useful and valuable in many decision-making scenarios, the wider and more comprehensive discipline of data science and analytics powers the next phase of decision-making — the ability to be predictive and prescriptive.
The Gartner Analytic Ascendency model visualises this evolution neatly. It identifies four different types of data analytics, reveals the dependency between them, and ranks them in terms of value — as well as difficulty.

Four Types of Data Analytics —Source: kdnuggets.com
Descriptive analytics answers the question “What happened?” This is traditional BI. The aim is to analyse historical data to provide, if you like, hindsight. For example, a retailer can learn how many sales were made last month, or a manufacturer how many products were returned.
Diagnostic analytics answers the question “Why did it happen?” It drills down into the descriptive data to find the cause or causes of the outcome — providing insight, in other words.
Predictive analytics helps organisations answer the question “What is likely to happen next?” It does so by analysing the findings from the first two types of analytics to predict future trends. Our manufacturer, for example, would use predictive analytics to forecast how many returns are likely to be made next month — and our retailer how many sales of which items are likely to be made to which customers based on last month’s (or last year’s/last season’s) trends.
Prescriptive analytics helps organisations answer the question “What do we need to do?” This is the most valuable type of data analytics. It combines the insights gained from all previous analyses to determine the course of action to take in the face of a current decision or problem — correct a defect to minimise returns to our manufacturer, for instance, or recommend which customers to target with a special offer or marketing campaign in the case of our retailer. In other words, rather than hindsight, predictive and prescriptive analytics take insights to give an organisation foresight.
The Challenges of Operationalising Data
Businesses the world over are indeed wide awake to the fact that they need to extract as much value as possible from the data they have in order to predict the future and gain competitive advantage. In fact, 95% of organisations surveyed for an IDG report last year said they have deployed big data initiatives on a department or enterprise level.
These organisations have wide-ranging goals — from improving decision-making to optimising the supply chain to redefining product development. As the report notes, business leaders know there’s hidden value within data, and expectations are high that capturing new insights from this data will unlock operational efficiencies and business growth.
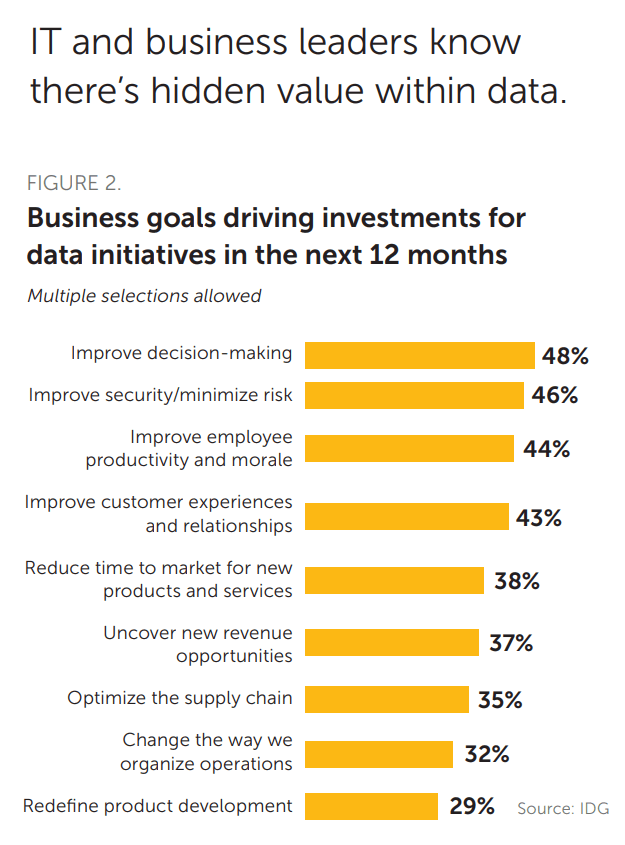
Nine Trends Shaping The Future Of Data Analytics —Source: arbelatech.com
However, despite the high expectations for business improvements, the same study reveals that the majority of organisations are struggling with delivering business outcomes from their data-driven programmes.
In fact, nearly two-thirds of respondents (64%) say that their organisations are using two or more analytics solutions to extract meaningful value from their data — and those solutions are often scattered across different parts of the business.
The result? 67% acknowledge that data analytics is “chaotic” at their organisation, and 70% agree that their data is underutilised.
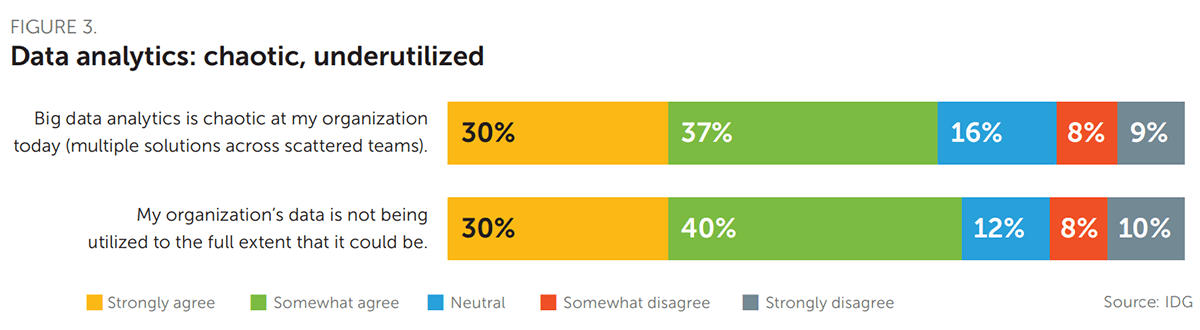
Nine Trends Shaping The Future Of Data Analytics —Source: arbelatech.com
Part of the problem, of course, comes down to the fact that the more organisations attempt to move from traditional BI towards data science — from descriptive and diagnostic analytics to predictive and descriptive — the more advanced the tools and skill sets need to be. Investments in AI and machine learning tools that use automated algorithms to churn through huge data sets, for example, are often considered necessary to operationalise data in predictive and prescriptive terms — and such tool sets can be prohibitively expensive for many scaling firms.
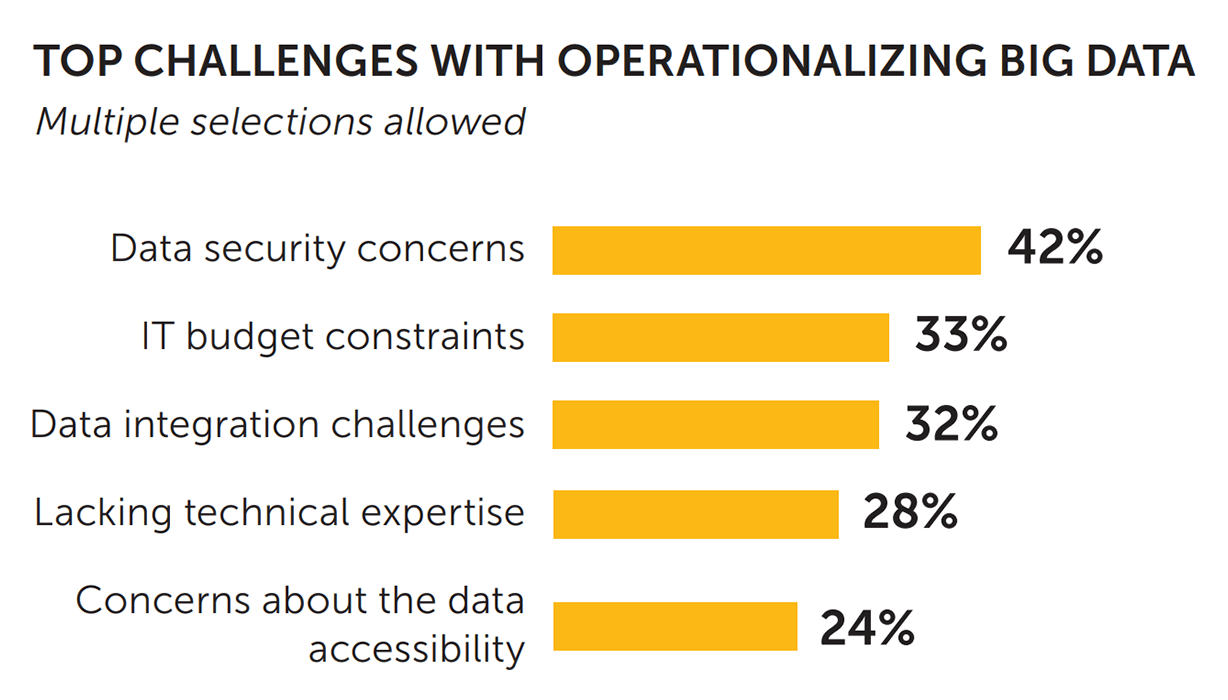
Nine Trends Shaping The Future Of Data Analytics —Source: arbelatech.com
At a more fundamental level, however, the sheer variety of data sources that cut across multiple databases, business systems, applications, individual business units and departments create significant challenges for organisations to start bringing order to the chaos and unearth the insights they need to create the foresight they desire.
As such, less than one-third of organisations (31%) say that they’re adept at extracting valuable insights from data across multiple sources to create trusted business assets — the rest consider themselves average at best.
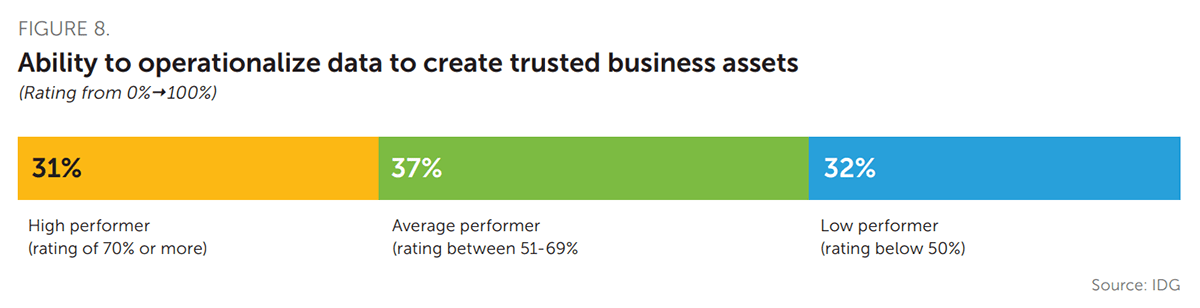
Nine Trends Shaping The Future Of Data Analytics —Source: arbelatech.com
Unlock a Goldmine of Data with metasfresh ERP
The truth is that many organisations won’t necessarily need complex algorithms running on their data to get started with data science (though such tools will certainly help, of course, especially when dealing with increasingly large data sets). What they do absolutely need, however, is easy access to their business data which is centralised in one place — and this is precisely where the value of a powerful ERP system like metasfresh is found.
Even the most experienced goldsmith can’t make a 22-carat ring from a 9-carat nugget. But when each business function of an organisation has its own hoard of department-specific data siloed away from everyone else’s — plus its own set of software tools that speak their own discrete languages — dusty piles of 9-karat nuggets is what they’re dealing with.
Open source ERP software like metasfresh, on the other hand, is an all-seeing, all-knowing system that integrates an organisation’s wide-spread business functions — from HR to finance, to accounting, to product development, to supply chain management, to sales, to marketing — into one, overarching information system.
Only by compiling an organisation’s entire hoard of multi-departmental business data in one place can meaningful data science happen. metasfresh is designed from the ground up to process mass data from across the organisation in order to help businesses make informed decisions and identify patterns that can be used for predicting future trends and prescribing future action — be it for balancing the supply vs. demand equation, responding quickly to market changes (such as during pandemics), or identifying when a certain department like customer support may require additional training to handle the barrage of questions about to flood in following a new product release.
All BI and data science initiatives — be they descriptive or prescriptive — begin by compiling, organising and enabling ready access to the raw data itself. metasfresh ERP is your single source of all business data upon which data science can thrive.
As Arun Ulag, Corporate Vice President at Microsoft, concludes in the IDG report: “More organisations are embracing a data culture that unifies information from many sources to drive business decisions. For a data-centric culture to thrive, it requires everyone to work from the same data platform and intuitive tools that let them leverage vast quantities of data quickly to reach insights.”
Talk to us here at metasfresh. Since 2006, we have been developing our metasfresh ERP software non-stop with open source components under the open source licenses GPLv2 and GPLv3. Our mission is to enable each and every company to access a powerful ERP system fuelled by data analytics to drive digital transformation and corporate growth. Get in touch today for more information and insights.
Share this Post